Apple Team Shared The Secret On How They Stay Ahead Of The Competition With A13 Chip
Aadhya Khatri
Phil Schiller and Anand Shimpi, two employees of Apple, shared their approach in the design of chips and how they improve the performance every year
- Apple Kills Original HomePod, Focusing On HomePod Mini
- iPhone 12 Color Is Fading Away Quickly And No One Knows Why
- Apple Sues Ex-Employee For Stealing Company’s Secrets To The Media
Recently, Phil Schiller, Apple’s VP of product marketing, and Anand Shimpi, a member of the Platform Architecture team, shared their approach in the design of chips and how they manage to improve their performance every year.
In their interview, they pointed out how they made the chip to become more power efficient and what users can gain from Apple’s effort.
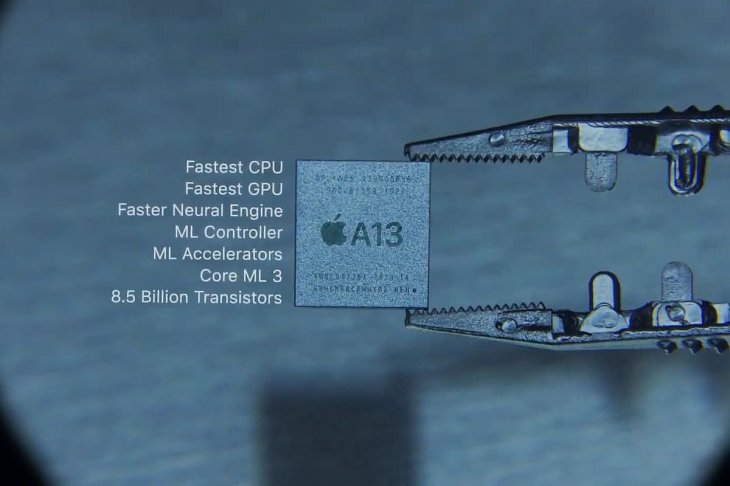
During the announcing event earlier this month, Apple shared some details regarding its A13 Bionic chip. It sports 8.5 billion transistors, two performance-focused cores, four efficiency-focused ones, making up a total of six cores, two machine learning accelerators, a quad-core GPU, and an octa-core neural engine. So the chip can work on a trillion operations each second.
So in comparison with the A12, the A13’s performance is 20% faster and its efficiency is increased by 30%. Many of Apple’s competitors now have eight cores in their chips, the iPhone maker’s smooth integration of software and hardware gives it a competitive edge over them all.
According to Anand Shimpi and Phil Schiller, their focus was efficiency when they develop the chip:
To shed some more light on the process of developing the chip, Schiller and Shimpi shared that CPU designs are guided by specific applications.
Apps that do not require further optimization will use less energy than usual. The interview also uncovered that the iPhone maker makes use of the same approach to develop its machine learning and GPU.
The fact that the A13 has a different way of processing has made it stand out from other chips on the market.
You can imagine the A13 to have one single home basis but with the usual on-and-off approach, which can help reduce the rate of electrodes going waste.
Schiller said that the key for all these optimizations was machine learning:
Featured Stories
Features - May 09, 2025
Huawei Officially Launches PC-Exclusive Operating System, Completely Replacing...
ICT News - Apr 09, 2025
Experts Say Trump's Call for US-Made iPhones Could Triple Prices
ICT News - Apr 08, 2025
How Would US Tariff Affect Tech Prices in India?
ICT News - Feb 26, 2025
Elon Musk's Federal Workforce Overhaul: AI Takes the Helm
ICT News - Feb 26, 2025
Will AI Kill Coding Jobs? The Truth Might Surprise You
ICT News - Feb 25, 2025
Not Radiation: What Is Causing the Strange Genetic Evolution of Chernobyl’s Dogs?
ICT News - Feb 25, 2025
Google to Phase Out SMS-Based Authentication Codes
ICT News - May 17, 2022
3 Reasons your privacy gets compromised online
ICT News - May 11, 2022
Apple Devices For Sale
ICT News - Apr 12, 2022